By Raghav Narayanswamy
Earlier today, there were a lot of fun photos on our website, “drawings” of birds in Singapore in place of real images of them. Consider yourself April fooled! These drawings were a little different from the ones we put up on April Fools’ two years ago… because we didn’t draw them – machines did. On a more serious note, AI tools have changed the way a lot of people do things, and we might not even be aware of all the ways in which AI is being used in our daily lives.
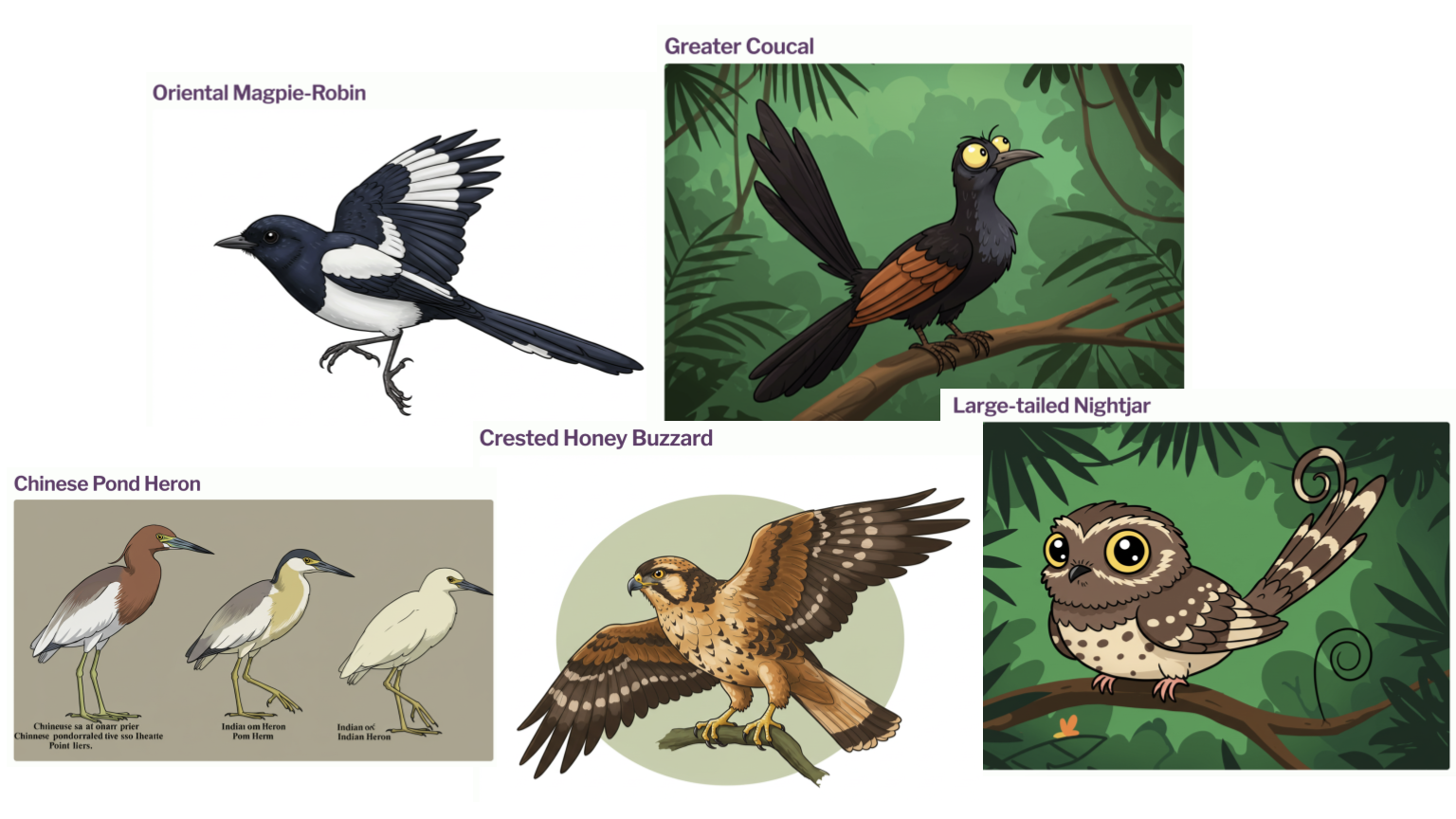
I want to emphasize four points at the start of this article.
- The April Fools’ prank of changing a limited number of bird images to AI generations highlights not just the fact that AI exists today and can be used to generate images, but it also shows the immense shortcomings of these models. These are the precise focus of this article, in fact, and we believe it is imperative that this is highlighted to as wide an audience as possible. It is immediately obvious that every AI-generated image that was uploaded on our site today is flawed in one way or another, even though none of the prompts asked for two-tailed birds, birds with four wings, or similar.
- Generating images using AI does not strengthen these models – if anything, they would be weakened by attempting to train on the low-quality outputs produced.
- Intellectual property is a very pressing issue with AI today. AI models have been trained on all kinds of copyrighted data, from art painstakingly crafted by artists, to entire novels, films, and documentaries. We do not aim to minimize this in the slightest, and it raises important points on both ethical and legal levels, as highlighted in this article. Yet, reality demands that we are aware of what the future holds – and as mentioned, we think it is important that attention is brought to the issues caused by AI in ensuring scientific data integrity, which is a widely under-recognized topic and the focus of this article. Again, this is why we highlight that the generated images are not life-like and poorly representative in one way or another, even though this was not asked for.
- On a slightly unrelated note, the environmental implications of AI models have been discussed extensively. The math for this isn’t clear, as highlighted in this article. The processors that power AI models are also getting twice as energy-efficient every 3-4 years.
The reality is that AI is here to stay, for better or for worse, and it is likely to change a lot of things that we know today. This article is specifically about how AI can affect how we keep track of bird records, which are important for influencing conservation. Singapore is also a place where there is always great interest among the birding community whenever a rare bird is seen; we all want to “twitch” the latest rare bird for ourselves, and some of us are also interested in bird identification. So these topics are very pertinent as they strike at how AI will change birding for all of us.
Malicious uses of AI
This part is straightforward. Tools which can generate realistic images can be used to create fake images of real birds. Reverse image searching to find the image online wouldn’t work because the image is newly generated. It’s also possible to falsely enhance images, for example, by adding a fake bird to an image with real birds in them – for example, taking a photo with two chicks in a nest and making it three.
Malicious uses of AI extend beyond what we are able to advise on – they are ultimately about people behaving dishonestly, and not a problem with the tool itself. If someone really wanted to fake a bird sighting, they could just take a photo taken overseas and claim it was taken in Singapore too. This happens, we have seen it, and we should keep looking out for it. But the focus of this article is more on how you might unknowingly fall into the traps of AI.
Seemingly harmless uses of AI
Image editing software like Photoshop and Lightroom have been around for years now, and many of us use these tools to enhance images. General image editing and finer-scale adjustments like branch removal are commonplace. But AI brings this to a different dimension.
One example is with object removal. Without AI, removing large objects in front of a bird is impossible – because ‘filling in’ the missing details is not possible without knowing what the bird should look like. But that still doesn’t mean that AI somehow knows what the bird should look like either! It doesn’t have X-ray vision to be able to see what’s behind the leaf in the image on the left below, for example:
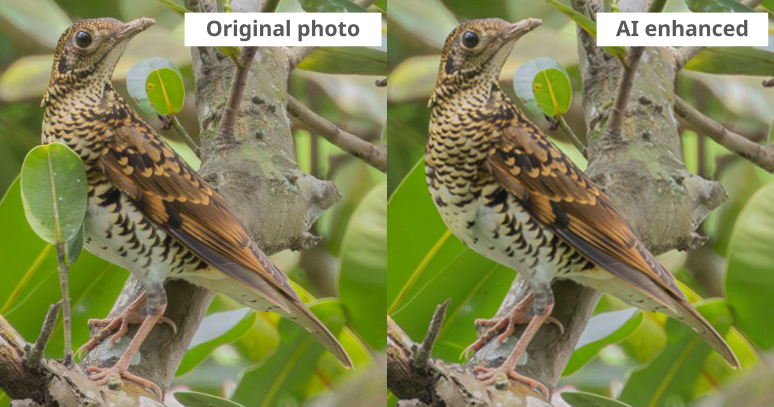
Yet the software is able to fill in the missing areas with something that looks reasonable, and it even generates realistic-looking, correctly-colored scales. It’s important to note that unlike non-AI-based editing tools, this is a generative process. When I ask my computer to remove the leaf, or maybe even replace it with something else like a small flower, there is something [1] that is actually generating what it thinks should be there. It tries to figure this out based on three main things:
- Its general knowledge of the world, or more specifically, whatever selection of the world it has been exposed to during the training process
- What it sees in the rest of the image
- My specific instructions (the “prompt”)
This all seems cool and nice, but it raises some important questions. How do I know that the model understands the rest of the image, and my prompt, in relation to what it should do with the region I’ve selected? For this specific example, does my computer know the bird is a White’s Thrush? Does it know what the belly of a White’s Thrush should look like? It certainly does not know what the belly of this White’s Thrush exactly looks like. Now a hypothetical: What if the specific area I’ve highlighted contains a feature separating two very similar-looking birds? The disconcerting answer to all of these questions is basically that no one knows. No one could tell you in advance what is going to happen for a certain image and prompt. Furthermore, because randomness is involved in the way these models work, the output will differ between two different executions of the same combination of prompt and image.
Now, it is not hard to see how this could be problematic. Basically anything that uses AI to edit images will apply its knowledge of the world, whatever that may be, to images which may not even fit its knowledge of the world to begin with. To make this less abstract, here are some more concrete, but still hypothetical, possibilities:
- Removing a distracting branch, like with the White’s Thrush above, could result in an Eastern Crowned Warbler ‘becoming’ an Arctic, if its crown stripe was blocked in the original image.
- Certain species might be overrepresented in the training data: most sparrowhawks a model has seen might be Eurasian, and most of its kingfishers might be Common. This kind of model would fail to generalize properly when faced with different species of sparrowhawk or kingfisher, and ‘convert’ species when attempting to merely enhance images.
- In some families of birds, many species have light eyes, so if the eye of a bird is shadowed in an image, attempting to just brighten it could result in the iris turning pale, even if it is not.
- Birds undergoing moult can look patchy or have unusual feather patterns. Attempting to ‘clean up’ or ‘enhance’ such an image might lead the AI to smooth over the moult pattern, filling it in with ‘complete’ feathering, thus inaccurately representing the bird’s actual condition at that moment. Because we use feather wear patterns to identify different birds of the same species, this might result in artificially inflated – or deflated – counts of rare birds.
- During upscaling, noisy areas may be interpreted as bars, or genuinely barred areas may be artificially smoothed. If this happens while other parts of the image maintain a high level of perceived detail, it might be impossible to tell whether the bird was really barred or not.
While these are all hypothetical, as mentioned, they are all perfectly conceivable. Particularly worrying is the fact that many models will have seen a lot of European and North American birds, and not many Asian ones. The same concept that applies to human faces, well illustrated by this widely circulated AI-upscaled image of Barack Obama, translates to all other kinds of images as well.
Here is an example of this from Imagen 3, an image diffusion model by Google. While this model differs from the AI image editing models mentioned above, because it has no base image to work from, the problems are still the same.
While it bravely attempts the white throat, and even seems to understand the dark brown flanks and vent of the White-throated Kingfisher, it is clear from the bird’s head and wings that the model badly wants every kingfisher to be from the genus Alcedo. It probably has seen too many Common Kingfishers in its life, and so its ‘Kingfisher World’ is hopelessly tied to the appearance of a Common Kingfisher.
No Stork-billed Kingfishers in Imagen 3’s ‘Kingfisher World’.
This is relevant with mobile phone photos too. Because generative AI is baked into modern mobile phones these days as a built-in software feature that often cannot be disabled, it’s impossible to say what kind of adjustments went into the photo that you see in your phone. There’s almost never a way to retrieve the original, unedited image anymore. We have to be really cautious when looking at photos from phones today, even if they’re taken with the help of binoculars or a scope. A Zappey’s Flycatcher just might turn into a Blue-and-white. For all we know even a Pale Blue Flycatcher could turn into a Blue-and-white.
Moving forward as a community
We’ve always had a small fraction of people who have published inaccurate data, intentionally or not. Photos taken elsewhere have been mistakenly or deliberately reported as being from Singapore, people have taken others’ photos and passed it off as their own. We’ve been able to identify some of these possible cases and especially for rare birds, we are careful when assessing records in the Singapore Bird Database.
AI already has, and will continue to, push the difficulty level a few notches up. Those who deliberately publish fake sightings are an extremely small minority. The difference with AI is that it is both powerful, in that it can be used to extensively improve images, but also dangerous in its ability to believably and reliably create what is not there.
Here are some of our suggestions for AI use, which we believe will benefit the birding community and ensure accurate records-keeping.
We should always declare if AI is used in uploaded photos, and how it is used. There is nothing to be ashamed about! When used well, tools like Photoshop’s Generative Fill, and even ‘simpler’ AI-powered tools like Topaz are extremely powerful. But for the reasons outlined in this article, we think any editing that involves AI should be highlighted in online posts. This will also help us learn more about how AI can affect – both positively and negatively – the photos we take. As I mentioned earlier, no one really knows what’s going on inside a neural network, but there might be some patterns we can find.
Keeping original, unedited photos (including RAW files, if available), will help with identification of difficult birds. This has always been the case but is even more true now. For rare birds, our Records Committee might request for these RAW files to help with identification. We don’t trust AI to stay faithful to the birds you photograph!
Our partner group, Bird Sightings, will be incorporating these guidelines into its group roles going forward as well. We hope this will move us towards a culture of openness and help us better understand how AI can continue to affect data and scientific integrity.
Oh, and this article? It was written by humans. And it’ll probably be used to train AI models too.
Acknowledgments
Different members of the Singapore Bird Records Committee contributed valuable comments to this article.
Footnote
[1] This is probably the first footnote on this website ever, and hopefully the last. While I should probably be a bit more specific than “something”, I don’t want to clutter the main text, so I’ll put it here: the “something” we speak of is a type of artificial intelligence called a deep neural network, essentially a complex pattern-matching system. It’s trained by showing it a huge number of example images, often paired with text descriptions. For the specific task of filling gaps (inpainting), the training often involves: taking an image, randomly masking a part of it (like covering it with a blackened area), and then asking the network to recreate the original hidden part. Crucially, it learns to do this using both the text description associated with the image and the visual information from the parts of the image it can still see. Over many, many examples, it gets remarkably good at generating pixels that fit both the description and the surrounding context. Here is an overview of how this process works – it’s slightly different and simpler in that it doesn’t include the text prompt part, but the general concept is similar.